Projects
Learning to recognize faces - The "Other-Race" Effect
Many people have a harder time recognizing faces that belong to categories they have little experience with. Faces of different ages, different races or ethnicities, or different species are harder to recognize and remember than the faces that we have the most experience with. In the Balas Lab, we study the development of this aspect of face learning by using looking time experiments and ERPs (event-related potentials). ERPs are recorded by putting a sensor net on a child's head (below left) and listening to what the brain does in response to different pictures or sounds.
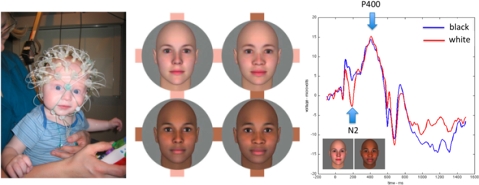
Here, you can see examples of how we use graphics software to manipulate the way different faces look. In this experiment, we asked how the shape and skin color of own- and other-race faces affect recognition independently. The faces in the middle either have matching shape and skin tone (top left and upper right) or mismatching shape and color (top right and lower left). We have found interesting differences in how the brain responds to other-race faces based on these properties.
Recognizing textures - pattern vision or "statistical" vision?
We recognize many things around us by examining exactly what shape features (edges, corners) we see in particular places. On the other hand, there's a whole class of recognition problems that seem to depend much less on knowing exactly where we saw something. Texture recognition is a perfect example of this, since the features that define a texture tend to scattered more or less uniformly over the entire image. In the Balas Lab, we're interested in figuring out just what problems you can solve by using texture processing and in collaboration with Ruth Rosenholtz at MIT, working out how much of your ordinary vision is actually forced to work by measuring summary-statistics of appearance.
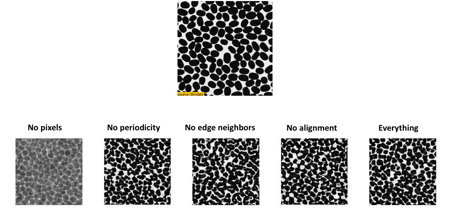
Above, you can see a texture of made up of coffee beans in silhouette and some synthetic textures we made from this image using a graphics model. By removing some information and leaving other things untouched, we can figure out what measurements you make to decide what a texture looks like and what properties it has.
Recognizing things that move
The visual world moves around us and we move through it. A critically important goal for the Balas Lab is to understand how object motion (e.g. facial gesture, body language) contributes to recognition. How do you recognize a person from the way that they walk? How does a facial movement convey emotion? We examine these questions by looking for invariant properties of dynamic visual recognition; How much does it matter if we play a sequence forward or backward? Does backward walking tell you less about someone than forward walking?

In the study pictured here (in collaboration with Nancy Kanwisher and Rebecca Saxe at MIT) we asked children to tell us if another child playing with Legos in a movie looked like they were playing alone or not. The trick? Kids only got to see a few seconds of video without sound, and edited to only show the target child on their side of the table. By asking kids to tell us what they can see in videos like these, we can understand how they make social decisions based on visual input.